How to Properly Find the IQR: A Practical Guide for Data Analysis in 2025
The interquartile range (IQR) is a fundamental concept in statistics, representing the range within which the middle fifty percent of your data lies. Understanding **how to find IQR** is crucial for effective data analysis, particularly for identifying outliers and studying data distributions. This guide offers a comprehensive approach, detailing the steps to calculate IQR, its importance in data analysis, and practical applications in various fields.
Understanding IQR: Definition and Importance
The **IQR definition** is fairly straightforward: it is the difference between the first quartile (Q1) and the third quartile (Q3) of a dataset. In essence, IQR tells us about the spread of the middle half of the data. By focusing on this central segment, IQR is less affected by extreme values, making it a **robust statistics** measure of variability. Understanding the significance of IQR allows analysts to accurately convey the data's nature and variability without being skewed by outliers.
IQR in Data Analysis
In data analysis, IQR plays a vital role in **descriptive statistics**. It helps in understanding data spread by providing insight into where the bulk of the data lies. For instance, in a study measuring test scores, utilizing **IQR for grouped data** allows you to see the performance levels without being misled by a few exceptionally high or low scores. Thus, it's invaluable for **analyzing data** and interpreting results in a meaningful way.
Applications of IQR in Various Fields
The **importance of IQR** extends far beyond simple statistics; it has applications in business, healthcare, and social sciences. For instance, researchers might use the IQR to determine patient outcomes or to assess financial performance metrics while making sense of variable score distributions. By employing the **IQR method** for **outlier detection with IQR**, businesses can identify irregular sales figures which could indicate fraud or errors. This enhances data interpretation and drives strategic decision-making.
Calculating IQR: Step-by-Step Guide
Knowing **how to compute IQR** is essential for conducting robust data analysis. The IQR is calculated using an easy-to-follow procedure. To provide a clearer understanding, let’s break down the process into simple steps, ensuring you can easily implement the **IQR formula** in various datasets.
1. Arranging Your Data
Begin by organizing your dataset in ascending order. This arrangement is necessary as quartile calculations rely on ordered values. For example, if your dataset is [3, 6, 7, 10, 15], it must be sorted as such. This step is crucial for accurate **interquartile range calculation**.
2. Finding Quartiles
Next, identify the first quartile (Q1) and third quartile (Q3): - **Q1** is the median of the lower half of the dataset (the first 50%). - **Q3** is the median of the upper half of the dataset (the last 50%). Suppose your dataset has 10 values; find the medians of the first five and the last five numbers. If these calculated quartiles yield Q1 = 6 and Q3 = 10, move to the next step.
3. Calculating the IQR
Utilize the following **IQR formula**: \[ \text{IQR} = Q3 - Q1 \] For instance, using Q1 = 6 and Q3 = 10: \[ \text{IQR} = 10 - 6 = 4 \] This gives you the IQR, which helps in understanding the data variance. It reflects the range in which the majority resides, hence is essential for **interquartile range significance**.
Principles of Using IQR for Outlier Detection
Using IQR for outlier detection is a practical application that many analysts leverage for cleaner data interpretations. To ascertain whether a data point is an outlier, you can use the following approach based on established statistical thresholds.
Identifying Outliers
Outliers are defined as those extreme values that lie beyond the range determined by the IQR. The thresholds can be calculated as follows: - Lower Threshold: \( Q1 - 1.5 \times \text{IQR} \) - Upper Threshold: \( Q3 + 1.5 \times \text{IQR} \) Any data points lying outside these thresholds are considered outliers. For example, if your calculated IQR = 4 and Q1 = 6, then: - Lower Threshold: \( 6 - (1.5 \times 4) = -6 \) - Upper Threshold: \( 10 + (1.5 \times 4) = 16 \) Thus, any data point below -6 or above 16 should be investigated further as potential outliers.
Visualizing IQR: Box Plots
One of the best ways to visualize IQR is through box plots. This visual representation provides an intuitive understanding of the data spread and highlights potential outliers effectively. In a box plot, the box itself represents the IQR, with lines—whiskers—extending to the minimum and maximum values within the thresholds. This **visualizing IQR** can be particularly powerful in presentations to convey statistical findings to wider audiences efficiently.
Key Takeaways
- The **IQR** measures the spread of the middle fifty percent of data.
- Calculating IQR requires sorting data and identifying Q1 and Q3.
- IQR is a robust measure that mitigates the influence of outliers.
- Using IQR for identifying outliers can improve data integrity and analysis.
- Visual tools like box plots facilitate better interpretation of data distributions.
FAQ
1. What is the basic definition of IQR?
The **IQR definition** is the range between the first quartile (Q1) and the third quartile (Q3) of a dataset. It measures the spread of the middle half of the data, providing insights into the data distribution by prioritizing this central segment, thus making it less affected by extreme values.
2. How is IQR computed for grouped data?
To calculate IQR for **grouped data**, ensure first to determine the cumulative frequency for your data groups. Then, derive Q1 and Q3 from these frequencies, utilizing appropriate formulas for grouped distributions. This approach is insightful for summarizing data fluctuations effectively.
3. Why is IQR preferred over standard deviation?
IQR is often preferred over standard deviation when dealing with **skewed data** or outliers. This is because while standard deviation takes every value into account, the IQR focuses solely on the center of the data, providing a more robust measure of data spread and variation.
4. Can I use IQR in trending analysis?
Yes, **analyzing data trends** using IQR can aid in understanding market dynamics, consumer behavior, or any data variation over time. The stability of the IQR across different datasets can point toward overall performance and trends without the distraction of extreme values.
5. What are some common mistakes when calculating IQR?
Common mistakes when calculating IQR include misidentifying quartiles due to poor dataset organization or overlooking data points when calculating Q1 and Q3. Ensuring a stepwise approach and careful verification against datasets avoids these errors, leading to more reliable results.
In conclusion, mastering the process of **how to find IQR** enhances your ability to conduct substantive data analysis and interpret variability effectively. With a solid grasp of the IQR methodology, you can apply these principles across various contexts, improving the accuracy and reliability of your results.
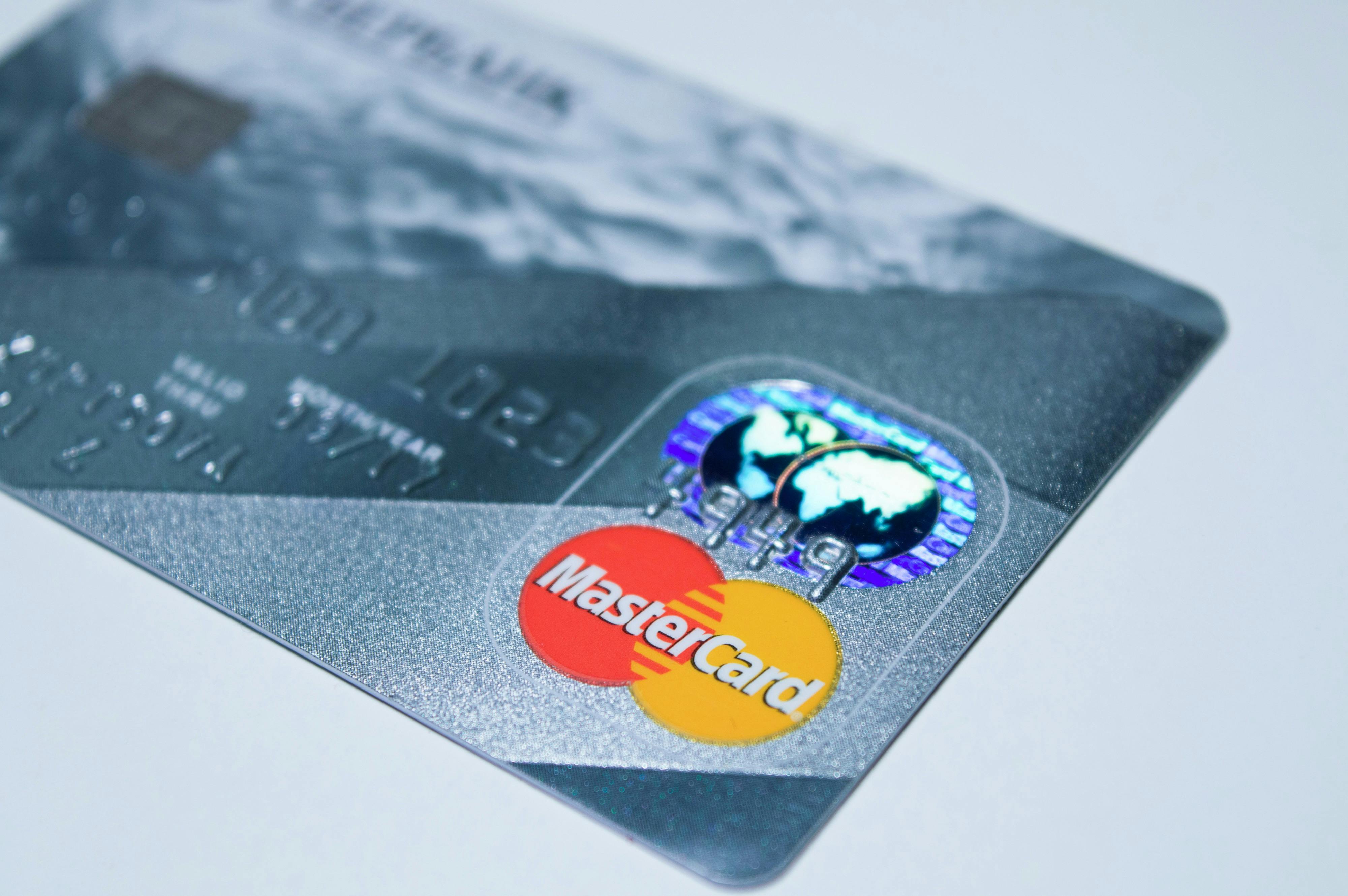
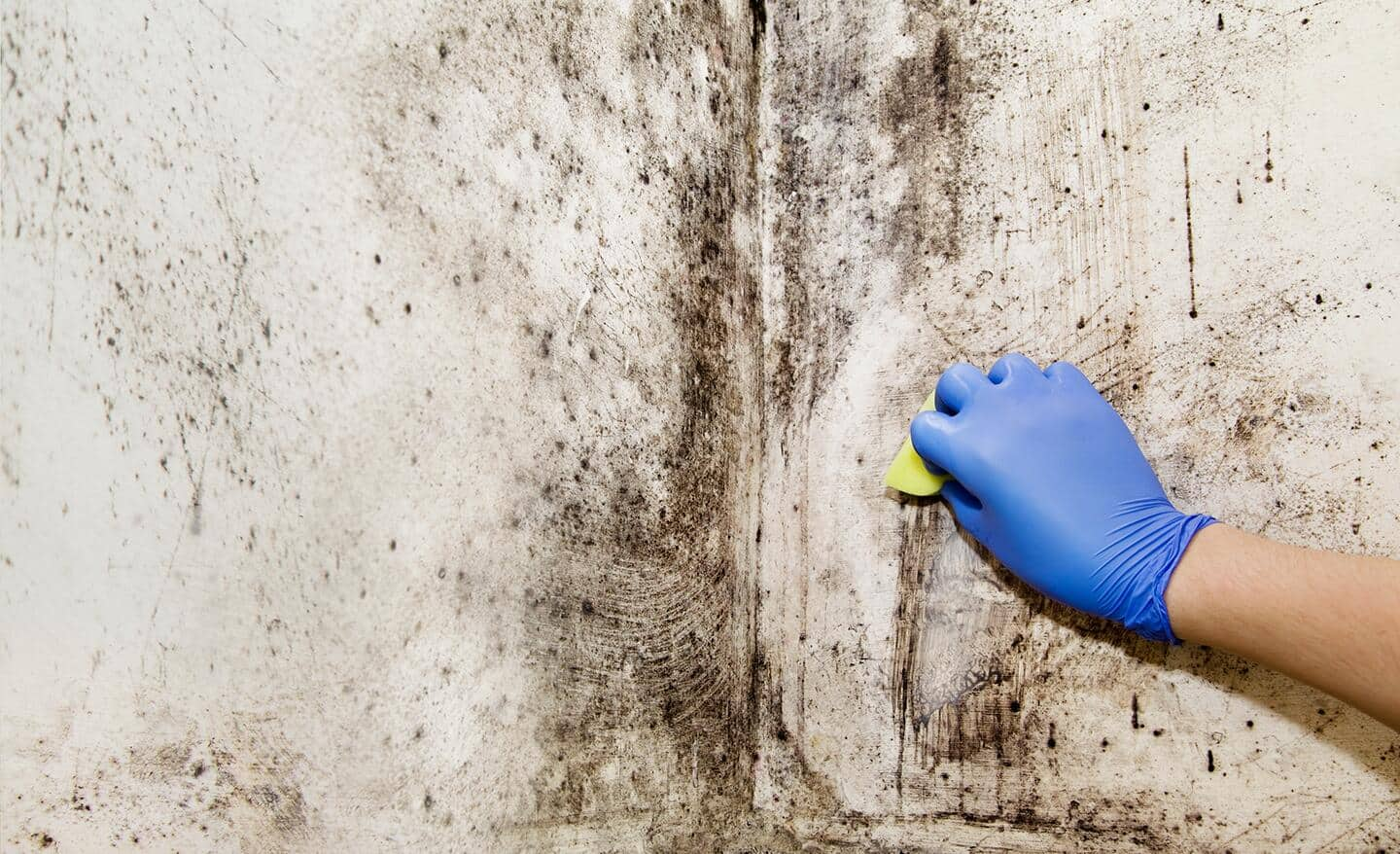